Starting with the hypothesis that analogical reasoning consists of a search of semantic space, weused eye-tracking to study the time course of information integration in adults in various formats ofanalogies. The two main questions we asked were whether adults would follow the same search strategies for different types of analogical problems and levels of complexity and how they would adapttheir search to the difficulty of the task. We compared these results to predictions from the literature.Machine learning techniques, in particular support vector machines (SVMs), processed the data to findout which sets of transitions best predicted the output of a trial (error or correct) or the type of analogy (simple or complex). Results revealed common search patterns, but with local adaptations to thespecifics of each type of problem, both in terms of looking-time durations and the number and types ofsaccades. In general, participants organized their search around source-domain relations that they generalized to the target domain. However, somewhat surprisingly, over the course of the entire trial, their search included, not only semantically related distractors, but also unrelated distractors, depending onthe difficulty of the trial. An SVM analysis revealed which types of transitions are able to discriminate between analogy tasks. We discuss these results in light of existing models of analogical reasoning.
Understanding the What and When of analogical reasoning across analogy formats: An eye-tracking and machine learning approach
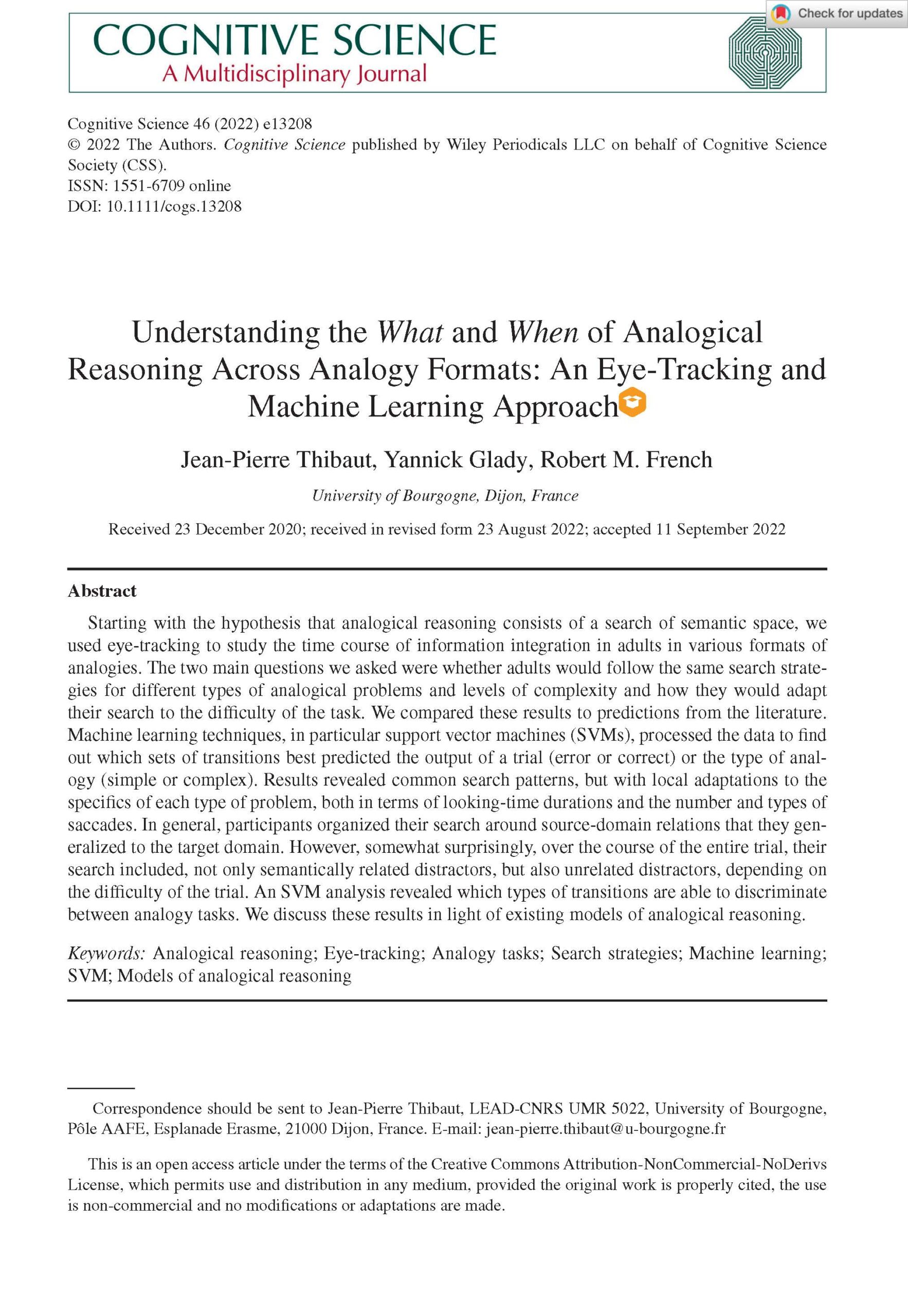