We propose a method for recruiting asymptomatic Amyloid positive individuals in clinical trials, using a two-step process. We first select during a pre-screening phase a subset of individuals which are more likely to be amyloid positive based on the automatic analysis of data acquired during routine clinical practice, before doing a confirmatory PET-scan to these selected individuals only. This method leads to an increased number of recruitments and to a reduced number of PETscans, resulting in a decrease in overall recruitment costs. We validate our method on three different cohorts, and consider five different classification algorithms for the pre-screening phase. We show that the best results are obtained using solely cognitive, genetic and socio-demographic features, as the slight increased performance when using MRI or longitudinal data is balanced by the cost increase they induce.We show that the proposed method generalizes well when tested on an independent cohort, and that the characteristics of the selected set of individuals are identical to the characteristics of a population selected in a standard way. The proposed approach shows how Machine Learning can be used effectively in practice to optimize recruitment costs in clinical trials.
Reduction of recruitment costs in preclinical AD trials: validation of automatic pre-screening algorithm for brain amyloidosis
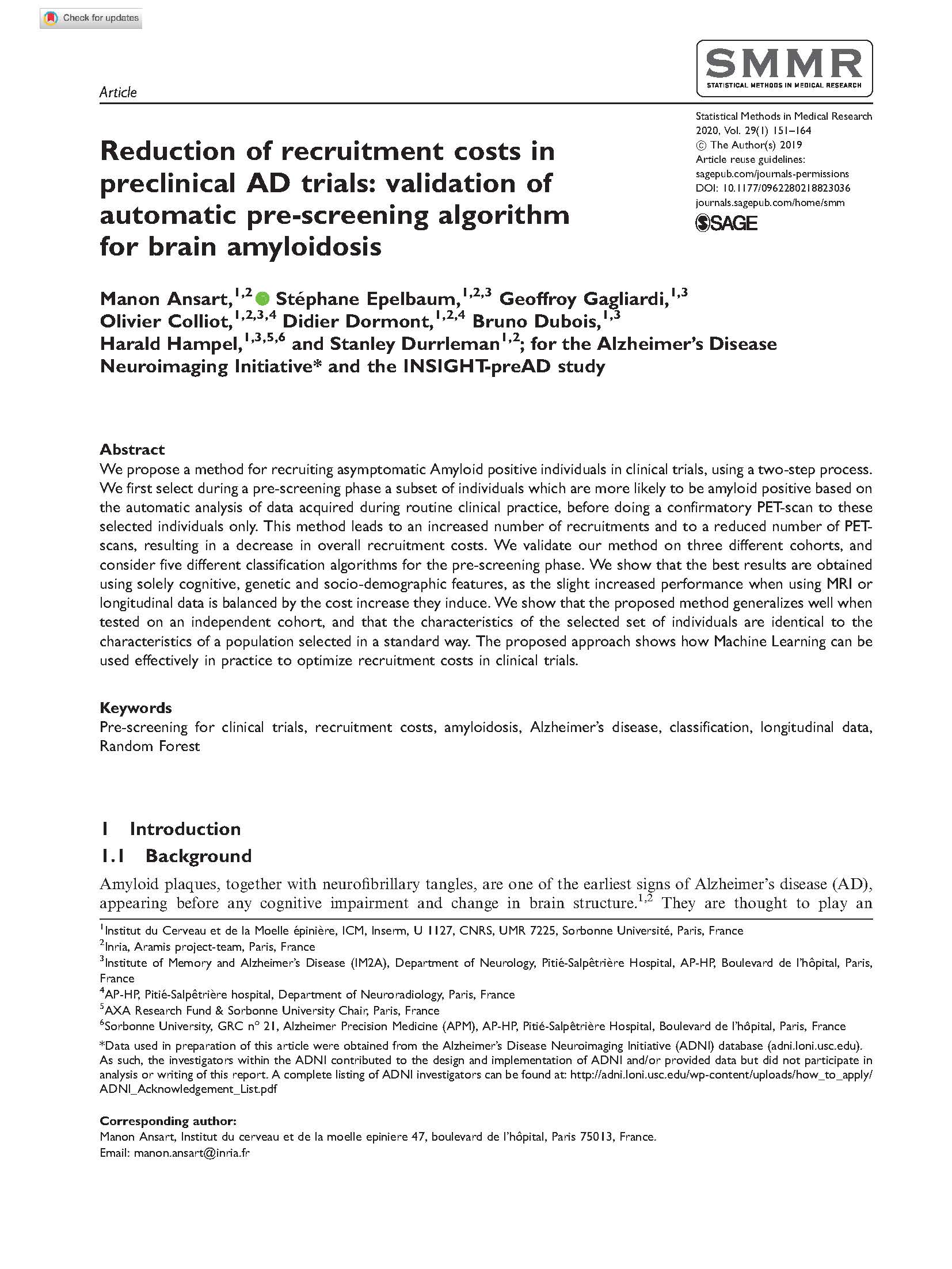